Jack Solowey and Jennifer Huddleston
California lawmakers’ heavy‐handed attempt to regulate cutting‐edge AI development (SB 1047) received appropriate attention and backlash from the pro‐innovation policy community. That backlash shone an important spotlight on the challenges state legislatures pose to AI innovation nationally.
Indeed, 40 states have considered some form of AI legislation this year alone, threatening to create an unworkable multi‐state patchwork. The initiatives range from laws targeting specific AI applications (such as AI’s use in music under Tennessee’s ELVIS Act) to regulatory regimes for AI broadly.
In addition to legislation like SB 1047 designed to tackle frontier AI risk, another category of relatively broad AI legislation worth paying close attention to seeks to regulate AI’s use in so‐called “consequential decisions,” such as employment, health care, and financial determinations.
While the risks these consequential‐decision acts seek to tackle are seemingly more mundane than the putative threats of AI‐enabled mass destruction targeted by frontier model legislation, the risks the consequential‐decision acts pose to AI development are by no means trivial. They threaten AI development by undermining naturally emerging business models, putting AI developers in jeopardy, and targeting technologies not just harms.
Consequential‐decision acts are gaining traction in the states. On May 17, Democratic Governor Jared Polis signed into law Colorado’s consequential‐decision bill, albeit under a bit of protest. With the Colorado bill advancing into law before even the EU’s AI Act, the typically innovation‐embracing state has come out ahead in a race it shouldn’t want to win.
On May 21, the California State Assembly passed its variation on the theme in a bill covering “Automated decision tools” (AB 2930), which is now before the California State Senate.
Though their details vary, both the Colorado and California consequential‐decision acts seek to combat the risks of AI decision tools perpetrating algorithmic discrimination (which is roughly defined to mean unlawful disparate treatment or impacts disfavoring people based on their membership in a protected class). Specifically, the acts seek to combat discrimination when AI tools are used for decisions that have material, legal, or similar effects on access to things like education, employment, housing, utilities, health care, legal services, and financial services.
The Colorado and California regulatory approach addresses potential discrimination through a suite of obligations placed to varying extents on AI decision tool developers and deployers (i.e., the organizations using the tools in interactions with consumers). The acts generally impose duties—differing in their particulars—to avoid algorithmic discrimination, perform risk assessments, notify individuals regarding the use of AI decision tools, provide consumers with rights to opt out of and/or appeal automated decisions and implement AI governance programs designed to mitigate the risks of algorithmic discrimination.
The Colorado and California acts’ automated decision opt‐out and appeal rights provide a window into the two regimes’ similarities, subtle differences, and ultimate problems. Whereas the California bill creates a new automated decision opt‐out right on top of an existing one mentioned in the state’s consumer privacy law, the Colorado law refers to a similar right in the state’s own privacy law while also adding new rights to appeal certain automated decisions.
Notably, California’s data privacy regulator has also begun preliminary rulemaking activity for California’s existing automated decision opt‐out right. This points to a broader conversation that must be had regarding the interactions between data privacy laws and AI. Existing privacy regulations may not be well‐adapted to the AI era. For instance, such laws’ data minimization requirements and limits on the use of personal information could undermine attempts to combat bias through more diverse data sets.
As for the opt‐out/appeal rights in the automated‐decision acts themselves, generally, both bills require some form of alternative decision process or human review when it’s requested by a consumer and is “technically feasible,” but Colorado would require the consumer to wait for an adverse decision, while California is less clear on timing.
There’s something superficially enticing to many about circumventing automated decisions, but creating a blanket right to do so is not without costs. Indeed, automation often will be precisely what provides the cost savings that allow a business to offer products or services at an attractive price. Mandated opt‐out rights likely would result in certain products and services becoming more expensive or unavailable.
Colorado’s more limited appeal right is a better approach but ultimately would impose similar costs, just to a potentially lesser degree. Furthermore, the caveat in the acts that alternative processes and human review be “technically feasible” is unlikely to help businesses with the technical ability to provide alternatives but without the resources to do so cost‐effectively.
Absent opt‐out mandates, businesses still would be able to provide such rights in response to consumer demand, while the broader ecosystem could simultaneously provide a greater range of features and prices.
The opt‐out mandates’ constraint on naturally emerging business models is one of the core issues with the Colorado and California proposals. The others are the legal jeopardy and compliance burdens imposed on AI developers, as well as regulatory approaches that target technologies instead of harms.
The Colorado and California consequential‐decision acts both impose onerous compliance risks and obligations on AI developers. Specifically, the acts inappropriately require developers, not just deployers, to anticipate and mitigate the risks of algorithmic discrimination. (Absurdly, the California bill even obligates AI developers to give legal advice to deployers, requiring developers to provide a “description of the deployer’s responsibilities under” the act.)
One major problem with this general approach is that it’s difficult, if not impossible, for a developer to completely understand an AI system’s propensity for discrimination in a vacuum or to predict every possible way their tool may be used. Any discriminatory effect of an AI system likely would be a product of both the underlying model and the deployer’s use, including the real‐world data the deployer feeds into the model at the inference stage, as well as the deployer’s ability to implement compensating controls addressing any disparate outputs.
One way the acts address this problem is by cabining some developer obligations to only those risks that are “reasonably foreseeable.” Nonetheless, the California bill undermines this limitation by imposing a general duty on developers to “not make available” an AI decision tool “that results in algorithmic discrimination.” While the Colorado law does a better job of limiting developer duties to only reasonably foreseeable risks, it nonetheless has unreasonable expectations regarding what developers will be able to predict and take responsibility for. The Colorado law mistakenly assumes developers will have greater knowledge of an AI tool’s “intended uses” than is likely to be the case and requires developers to notify law enforcement after discovering their tool’s use by a deployer is likely to have caused algorithmic discrimination.
Requiring developers to orient their compliance measures around predicted use cases risks limiting the types of productive ends to which their models may be applied, as novel use cases could increase compliance risk. Disincentivizing developers from allowing all but the most obvious intended uses would be a huge loss for the AI ecosystem, as some of the most creative applications of technologies typically are devised downstream from the tool’s creator. That’s why, for example, third‐party apps exist for smartphones.
Perhaps the original sin of the consequential‐decision acts is that they target AI used for, well, consequential decisions. Such decisions tend to be those related to sectors that already are heavily regulated, such as health care and finance. For example, the core risk addressed by these acts—discrimination based on protected class membership—already is illegal in credit decisions under federal law. Targeting the technology, as opposed to the harm, in the financial services context, for instance, is redundant at best and counterproductive at worst, as it adds yet another layer of compliance burden that could stymie AI tools’ potential to expand credit access to the historically underserved. In addition, this general approach often misassigns the blame for bad or negligent actors’ improper use of technology to the technology itself.
This shortsighted regulatory playbook—constraining business models, burdening developers with responsibility for downstream risks, and targeting technologies instead of harms—is being employed all too often at the state level. After all, SB 1047 is a notorious vehicle for all three, making open‐source AI development a compliance risk by requiring developers to lock down their models against certain downstream modifications, as well as targeting technical sophistication, not merely specific threats.
The risk from this playbook is that the US will be made worse off as state‐level frameworks become de facto national standards without the benefit of national input. This is not just the case for legislation out of large states like California, as laws with long‐arm ambitions and cloud‐based targets can, in practice, extend compliance burdens beyond state borders. Where that’s the case, conflicting obligations and subtle variations can raise the question of whether full‐scale compliance is even possible.
Instead of seeking to be the first to regulate, states should consider working from an alternative playbook that prioritizes innovation, avoids counterproductive interventions, and targets harms, not technologies. In the meantime, we should fear the words, “I’m from the state government, and I’m here to help with AI risk,” even when it’s another state’s government saying them.
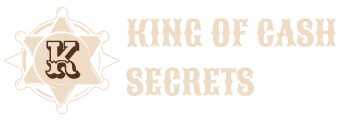
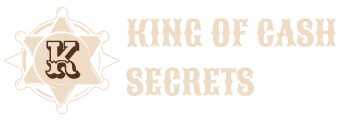